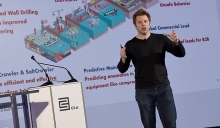
Daniel Jeavons
General Manager, Data Science, Royal Dutch Shell
Data to Decisions
Shell has been an early adopter of artificial intelligence (AI), as it attempts to speed up its digital transformation and energy future. From machine learning to computer vision, deep learning to virtual assistants and autonomous vehicles to robotics, Shell has been focused on a range of technologies that have supported advances in AI. Shell.ai was formed to provide a center of excellence to drive a common approach to data science technology solutions, demonstrate the art of the possible, and share best practices across the entire business.
Shell is a full stream energy company going through a significant transition to support the world’s need for more sustainable energy. That places a wide range of needs on different aspects of the business, from exploration and production, to distribution and trading, to retail and customer experience. The Shell.ai story is about taking a large organization with over 80,000 employees through digital transformation. Our ambition is to transform Shell into a natively digital company with tools and data widely available to generate insights. However, this transition is hard. On the one hand, we have to make our people passionate about solving their biggest problems with data; while on the other hand, we need to rapidly scale solutions to the entirety of Shell. When we give citizen data scientists the low-end tools to solve problems themselves, they come up with incredibly creative solutions, and they become convinced of the value of a data-driven solution. At the same time, dealing with the consistency and sustainability of a single, standardized approach and platform becomes necessary to actually deploy a solution at scale to cover Shell’s assets globally. The challenge is doing both these at the same time.
The Shell Digital team worked with a refinery technical monitoring team to design a data-based solution to monitor a valve remotely and predict fouling and failure. We scaled this ourselves to a few hundred valves. However, if you want to monitor 500,000 valves with machine learning algorithms, where not every valve is the same and needs a few of its own models, you will soon have two million models to create, deploy, monitor, retrain, dynamically swap out, all at global scale. We recognized that this complexity was going to require a level of technology sophistication. We threw the challenge out to find someone who could show us a platform that could manage two million machine learning models at scale automatically. We didn’t believe it could be done, but C3.ai surprised us, and it’s been a game-changer to our effectiveness and efficiency.
With this solution, refinery operators suddenly go from receiving hundreds and thousands of noisy alerts to a few very predictive early warnings that direct their maintenance actions. The engineers who use this tool are excited, not just with the potential efficiency gains and safety improvements and cost savings, but also about what else they could do with the same approach – how about monitoring a million instrumentation reads in a refinery…this is game-changing in terms of our safe operations. It makes our plants more reliable. Importantly, it also plays into this digital journey for the entire company - because this is what prevents leaks, it’s also what reduces greenhouse gas emissions, and this is massive not just for our business but also for the planet. We can have a material impact on the way in which we do work across our business, and most important, we can have a massive impact on our collective energy future. And this first success is starting to infect the organization to come up with and request their own solution for their problem.
Shell is leveraging the C3 AI Suite as part of a strategy to scale AI applications across its global operations, spanning assets across more than 20 refineries, 25,000 oil and gas wells, and 40,000 service stations. Ultimately, we want to make sure our assets run continually and safely, that’s the core, so predictive maintenance is a critical part of that. A lot of that has to do with equipment failure, equipment like valves and compressors, so what we’re trying to do is deploy this over 500,000 valves globally, that means probably running in excess of over 2M machine learning models in production. That’s a big problem and that is where C3.ai supports us. If we can predict that in advance, and we know we can, and we can deploy that at scale across all of our assets, we would be able to reduce our deferment potentially by several percentage points, and that has a massive impact economically on the performance of the company.
Number of machines controlled/managed: Over 600,000 valves, wells, pumps, motors, and compressors
Number of sensors involved: Over a million sensors across all of Shell's global footprint
Data volumes processed: Over 70PBs of data; almost 1TB/month
Other significant measures: Over 10 source systems (or variants across geographies)
Using five years of historical time series data from valve sensors plus additional relational data from other source systems, we created a unified object model using the C3 AI Suite to represent the asset hierarchy, work order notifications, ML models, alert management, and time series data. We also built a UI and application workflow to allow end users to effectively manage the lifecycle of thousands of individual machine learning models and investigate anomalies and potential failures.
Shell formed a data science center of excellence to centralize a coordinated effort to deploy advanced analytics in all aspects of the energy business, from upstream to midstream and downstream, and even retail. Dan Jeavons was appointed general manager for data science, leading the CoE and reporting into the business side of the organization to ensure successful adoption of the technology. Dan has been a catalyst internally and an evangelist externally to promote Shell’s progress in this digital transformation.
Key innovations:
- Select foundational technologies to shift from descriptive and diagnostic analytics to predictive, prescriptive, and AI-driven insights
- Showcase what is possible with those technologies, especially evolving from traditional business intelligence and analytics to realtime advanced analytics
- Facilitate the sharing of best practices across Shell
Shell also separated innovation from operational support by creating a separate business service center to handle ongoing operational management and maintenance of advanced analytics deployments so the CoE could focus on innovation and alignment.
Our biggest challenge is taking successful pilots and scaling them into production where we have to operate and maintain them efficiently. We created a successful digital twin for 10 valves, then 100, but we needed to get to much larger scale to support a production environment. We’ve already proven we can handle the 160,000 valves at our Pernis facility, driving predictive maintenance savings that could mount into the hundreds of millions of dollars of economic benefit.
About Your Organization
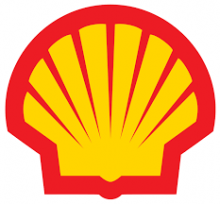
Royal Dutch Shell plc companies have operations in more than 70 countries and territories with businesses including oil and gas exploration and production; production and marketing of liquefied natural gas and gas to liquids; manufacturing, marketing and shipping of oil products and chemicals and renewable energy projects. For more information, visit www.shell.com.